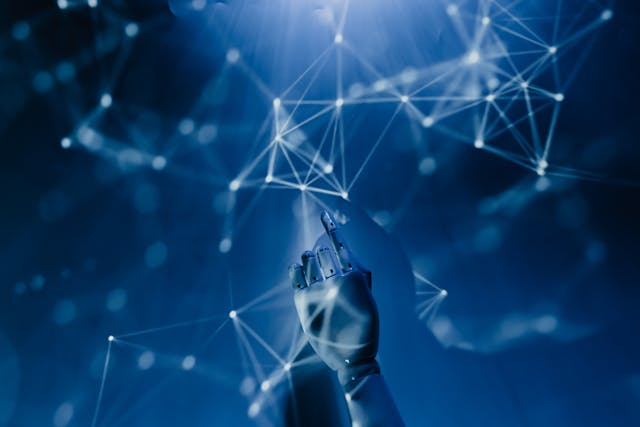
Generative AI machine learning systems capable of producing new material and artifacts including text, pictures, audio, and video are called generative artificial intelligence (AI). Large datasets are used to train generative AI models on patterns and their ability to produce fresh outputs depending on their learning. Though generative AI research began in the 1950s, access to huge datasets and developments in deep learning have caused it to grow in recent years.
Among the well-known instances of generative AI systems today are voice synthesis models like Whisper and WaveNet for audio production, big language models like GPT-4, DALL-E for image generation, Stable Diffusion, and Google Images. Although generative AI skills have advanced quickly, there are now fascinating new applications, which have also sparked worries about possible hazards and difficulties.
Generative AI Risks of Misuse
There are risks and challenges despite the many possibilities and benefits of AI. One major concern is the potential to spread misinformation and deepfakes on a large scale. Synthetic media makes it easy to generate fake news articles, social media posts, images, and videos that look authentic but contain false or manipulated information.
Related to this is the risk of fraud through impersonation. Generative models can mimic someone’s writing style and generate convincing text or synthesized media pretending to be from a real person.
Generating dangerous, unethical, illegal, or abusive content is also risky. AI systems lack human values if prompted and may produce harmful, graphic, or violent text/media. More oversight is needed to prevent the unchecked creation and spread of unethical AI creations.
Additional risks include copyright and intellectual property violations. Media synthesized from copyrighted works or a person’s likeness may violate IP protections. Generative models trained on copyrighted data could also lead to legal issues around data usage and ownership.
Bias and Representation Issues
Generative AI models are trained on vast amounts of text and image data scraped from the internet. However, the data used to train these models often lacks diversity and representation. This can lead to bias and exclusion in the AI’s outputs.
One major problem is the lack of diverse training data. An artificial intelligence model will struggle to produce high-quality outputs with different demographics if it is mostly trained on pictures of white individuals or text written from a Western cultural viewpoint. The data does not adequately represent the full diversity of human society.
Relying on internet data also means generative AI models often learn and replicate societal stereotypes and exclusions present online. For example, DALL-E has exhibited gender bias by portraying women in stereotypical roles. Without careful monitoring and mitigation, generative AI could further marginalize underrepresented groups.
Legal and Ethical Challenges
The rise of generative AI brings new legal and ethical challenges that should be carefully considered. A key issue is copyright and ownership of content. When AI systems are trained on vast datasets of copyrighted material without permission, and generate new works derived from that training data, it creates thorny questions around legal liability and intellectual property protections. Who owns the output – the AI system creator, the training data rights holders, or no one?
Another concern is proper attribution. If AI-generated content does not credit the sources it was trained on, it could constitute plagiarism. Yet existing copyright law may not provide adequate protections or accountabilities as these technologies advance. There is a risk of legal gray areas that allow misuse without technical infringement.
The AI system creators may also face challenges around legal liability for harmful, biased, or falsified content produced by the models if governance mechanisms are lacking. Generative models that spread misinformation, exhibit unfair biases or negatively impact certain groups could lead to reputation and trust issues for providers. However, holding providers legally responsible for all possible AI-generated content presents difficulties.
There are also emerging concerns around transparency and accountability of generative AI systems. As advanced as these models are, their inner workings remain “black boxes” with limited explainability. This opacity makes it hard to audit them for bias, accuracy, and factuality. A lack of transparency around how generative models operate could enable harmful applications without recourse.
Regulatory Approaches
The rapid advancement of generative AI has sparked debate around the need for regulation and oversight. Some argue that the technology companies developing these systems should self-regulate and be responsible for content moderation. However, there are concerns that self-regulation may be insufficient, given the potential societal impacts.
Many have called for government regulations, such as labeling requirements for AI-generated content, restrictions on how systems can be used, and independent auditing. However, excessive regulations also risk stifling innovation.
An important consideration is content moderation. AI systems can generate harmful, biased, and misleading content if not properly constrained. Moderation is challenging at the enormous scale of user-generated content. Some suggest using a hybrid approach of automated filtering combined with human review.
The large language models underpinning many generative AI systems are trained on vast datasets scraped from the internet. This can amplify harmful biases and misinformation. Possible mitigations include more selective data curation, techniques to reduce embedding bias, and allowing user control over generated content styles and topics.
Technical Solutions
There are several promising technical approaches to mitigating risks with generative AI while maintaining the benefits.
Improving AI Safety
Researchers are exploring techniques like reinforcement learning from human feedback and scalable oversight systems. The goal is to align generative AI with human values and ensure it behaves safely even when given ambiguous instructions. Initiatives like Anthropic and the Center for Human-Compatible AI are pioneering safety-focused frameworks.
Bias Mitigation
Removing harmful biases from training data and neural networks is an active area of research. Techniques like data augmentation, controlled generation, and adversarial debiasing are showing promise for reducing representation harms. Diverse teams and inclusive development processes also help create fairer algorithms.
Watermarking
Embedding imperceptible digital watermarks into generated content can verify origins and enable authentication. Startups like Anthropic are developing fingerprinting to distinguish AI-created text and media. If adopted widely, watermarking could combat misinformation and ensure proper attribution.
Conclusion
Generative AI has enormous potential but poses significant risks if used irresponsibly. Potential neglect, representation, and prejudice problems, moral and legal issues, and upsetting effects on business and education are some of the main obstacles.
While generative models can produce human-like content, they lack human ethics, reasoning, and context. This makes it critical to consider how these systems are built, trained, and used. Companies developing generative AI have a responsibility to proactively address the dangers of misinformation, radicalization, and deception.
The goal should be developing generative AI that augments human capabilities thoughtfully and ethically. With a comprehensive, multi-stakeholder approach focused on responsibility and human benefit, generative AI can be guided toward an optimistic future.
- SEO Powered Content & PR Distribution. Get Amplified Today.
- PlatoData.Network Vertical Generative Ai. Empower Yourself. Access Here.
- PlatoAiStream. Web3 Intelligence. Knowledge Amplified. Access Here.
- PlatoESG. Carbon, CleanTech, Energy, Environment, Solar, Waste Management. Access Here.
- PlatoHealth. Biotech and Clinical Trials Intelligence. Access Here.
- Source: https://www.theenvironmentalblog.org/2024/06/risks-challenges-generative-ai/